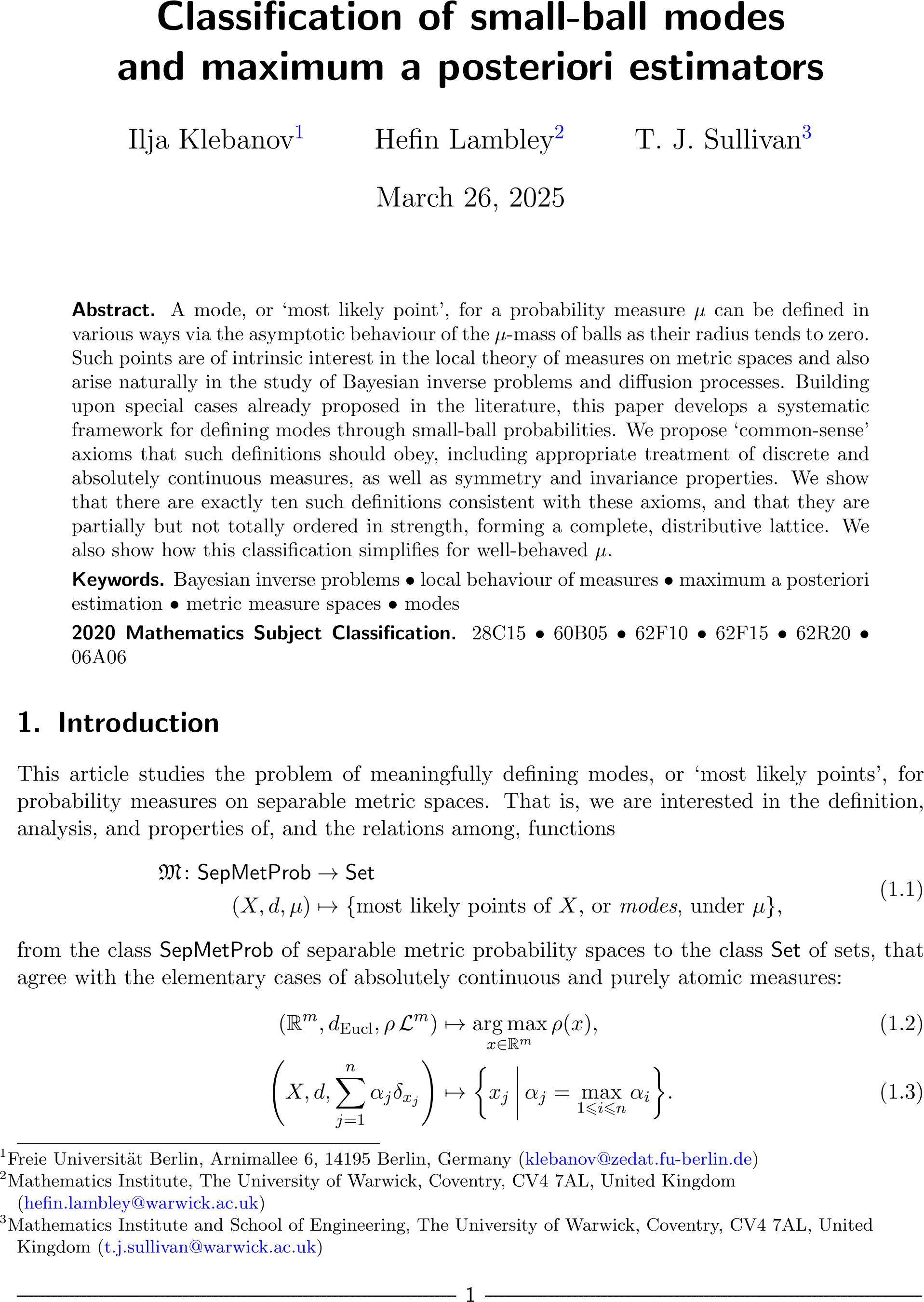
Classification of small-ball modes and maximum a posteriori estimators
Ilja Klebanov, Hefin Lambley, and I have just uploaded a preprint of our paper “Classification of small-ball modes and maximum a posteriori estimators” to the arXiv. This work thoroughly revises and extends our earlier preprint “A ‘periodic table’ of modes and maximum a posteriori estimators”.
Abstract. A mode, or “most likely point”, for a probability measure \(\mu\) can be defined in various ways via the asymptotic behaviour of the \(\mu\)-mass of balls as their radius tends to zero. Such points are of intrinsic interest in the local theory of measures on metric spaces and also arise naturally in the study of Bayesian inverse problems and diffusion processes. Building upon special cases already proposed in the literature, this paper develops a systematic framework for defining modes through small-ball probabilities. We propose “common-sense” axioms that such definitions should obey, including appropriate treatment of discrete and absolutely continuous measures, as well as symmetry and invariance properties. We show that there are exactly ten such definitions consistent with these axioms, and that they are partially but not totally ordered in strength, forming a complete, distributive lattice. We also show how this classification simplifies for well-behaved \(\mu\).
Published on Wednesday 26 March 2025 at 12:00 UTC #preprint #modes #map-estimators #klebanov #lambley