#ayanbayev
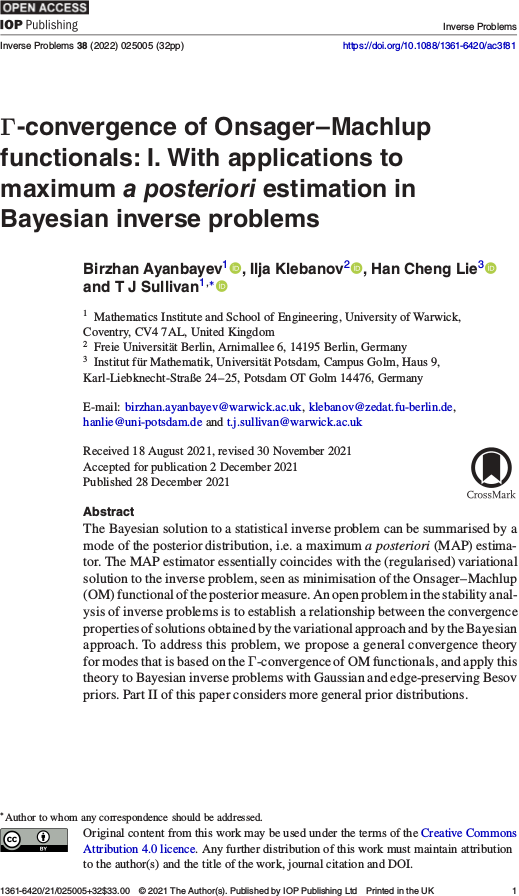
Γ-convergence of Onsager-Machlup functionals in Inverse Problems
The articles “Γ-convergence of Onsager–Machlup functionals” (“I. With applications to maximum a posteriori estimation in Bayesian inverse problems” and “II. Infinite product measures on Banach spaces”) by Birzhan Ayanbayev, Ilja Klebanov, Han Cheng Lie, and myself have just appeared in their final form in the journal Inverse Problems.
The purpose of this work is to address a long-standing issue in the Bayesian approach to inverse problems, namely the joint stability of a Bayesian posterior and its modes (MAP estimators) when the prior, likelihood, and data are perturbed or approximated. We show that the correct way to approach this problem is to interpret MAP estimators as global weak modes in the sense of Helin and Burger (2015), which can be identified as the global minimisers of the Onsager–Machlup functional of the posterior distribution, and hence to provide a convergence theory for MAP estimators in terms of Γ-convergence of these Onsager–Machlup functionals. It turns out that posterior Γ-convergence can be assessed in a relatively straightforward manner in terms of prior Γ-convergence and continuous convergence of potentials (negative log-likelihoods). Over the two parts of the paper, we carry out this programme both in generality and for specific priors that are commonly used in Bayesian inverse problems, namely Gaussian and Besov priors (Lassas et al., 2009; Dashti et al., 2012).
B. Ayanbayev, I. Klebanov, H. C. Lie, and T. J. Sullivan. “Γ-convergence of Onsager–Machlup functionals: I. With applications to maximum a posteriori estimation in Bayesian inverse problems.” Inverse Problems 38(2):025005, 32pp., 2022.
Abstract (Part I). The Bayesian solution to a statistical inverse problem can be summarised by a mode of the posterior distribution, i.e. a MAP estimator. The MAP estimator essentially coincides with the (regularised) variational solution to the inverse problem, seen as minimisation of the Onsager–Machlup functional of the posterior measure. An open problem in the stability analysis of inverse problems is to establish a relationship between the convergence properties of solutions obtained by the variational approach and by the Bayesian approach. To address this problem, we propose a general convergence theory for modes that is based on the Γ-convergence of Onsager–Machlup functionals, and apply this theory to Bayesian inverse problems with Gaussian and edge-preserving Besov priors. Part II of this paper considers more general prior distributions.
B. Ayanbayev, I. Klebanov, H. C. Lie, and T. J. Sullivan. “Γ-convergence of Onsager–Machlup functionals: II. Infinite product measures on Banach spaces.” Inverse Problems 38(2):025006, 35pp., 2022.
Abstract (Part II). We derive Onsager–Machlup functionals for countable product measures on weighted \(\ell^{p}\) subspaces of the sequence space \(\mathbb{R}^\mathbb{N}\). Each measure in the product is a shifted and scaled copy of a reference probability measure on \(\mathbb{R}\) that admits a sufficiently regular Lebesgue density. We study the equicoercivity and Γ-convergence of sequences of Onsager–Machlup functionals associated to convergent sequences of measures within this class. We use these results to establish analogous results for probability measures on separable Banach or Hilbert spaces, including Gaussian, Cauchy, and Besov measures with summability parameter \( 1 \leq p \leq 2 \). Together with Part I of this paper, this provides a basis for analysis of the convergence of maximum a posteriori estimators in Bayesian inverse problems and most likely paths in transition path theory.
Published on Wednesday 5 January 2022 at 12:00 UTC #publication #inverse-problems #modes #map-estimators #ayanbayev #klebanov #lie
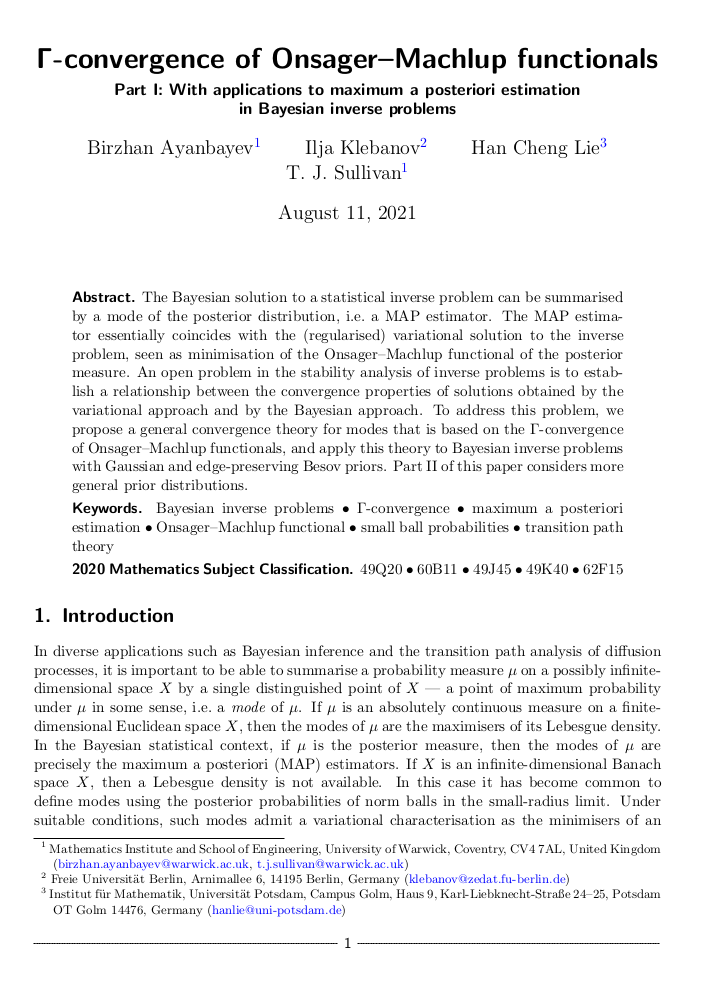
Γ-convergence of Onsager-Machlup functionals
Birzhan Ayanbayev, Ilja Klebanov, Han Cheng Lie, and I have just uploaded preprints of our work “Γ-convergence of Onsager–Machlup functionals” to the arXiv; this work consists of two parts, “Part I: With applications to maximum a posteriori estimation in Bayesian inverse problems” and “Part II: Infinite product measures on Banach spaces”.
The purpose of this work is to address a long-standing issue in the Bayesian approach to inverse problems, namely the joint stability of a Bayesian posterior and its modes (MAP estimators) when the prior, likelihood, and data are perturbed or approximated. We show that the correct way to approach this problem is to interpret MAP estimators as global weak modes in the sense of Helin and Burger (2015), which can be identified as the global minimisers of the Onsager–Machlup functional of the posterior distribution, and hence to provide a convergence theory for MAP estimators in terms of Γ-convergence of these Onsager–Machlup functionals. It turns out that posterior Γ-convergence can be assessed in a relatively straightforward manner in terms of prior Γ-convergence and continuous convergence of potentials (negative log-likelihoods). Over the two parts of the paper, we carry out this programme both in generality and for specific priors that are commonly used in Bayesian inverse problems, namely Gaussian and Besov priors (Lassas et al., 2009; Dashti et al., 2012).
Abstract (Part I). The Bayesian solution to a statistical inverse problem can be summarised by a mode of the posterior distribution, i.e. a MAP estimator. The MAP estimator essentially coincides with the (regularised) variational solution to the inverse problem, seen as minimisation of the Onsager–Machlup functional of the posterior measure. An open problem in the stability analysis of inverse problems is to establish a relationship between the convergence properties of solutions obtained by the variational approach and by the Bayesian approach. To address this problem, we propose a general convergence theory for modes that is based on the Γ-convergence of Onsager–Machlup functionals, and apply this theory to Bayesian inverse problems with Gaussian and edge-preserving Besov priors. Part II of this paper considers more general prior distributions.
Abstract (Part II). We derive Onsager–Machlup functionals for countable product measures on weighted \(\ell^{p}\) subspaces of the sequence space \(\mathbb{R}^\mathbb{N}\). Each measure in the product is a shifted and scaled copy of a reference probability measure on \(\mathbb{R}\) that admits a sufficiently regular Lebesgue density. We study the equicoercivity and Γ-convergence of sequences of Onsager–Machlup functionals associated to convergent sequences of measures within this class. We use these results to establish analogous results for probability measures on separable Banach or Hilbert spaces, including Gaussian, Cauchy, and Besov measures with summability parameter \( 1 \leq p \leq 2 \). Together with Part I of this paper, this provides a basis for analysis of the convergence of maximum a posteriori estimators in Bayesian inverse problems and most likely paths in transition path theory.
Published on Wednesday 11 August 2021 at 12:00 UTC #preprint #inverse-problems #modes #map-estimators #ayanbayev #klebanov #lie
Birzhan Ayanbayev joins the UQ Group
It is a pleasure to announce that Birzhan Ayanbayev will join the UQ research group as a postdoctoral researcher with effect from 28 February 2020. He will be working on the DFG-funded project “Analysis of maximum a posteriori estimators: Common convergence theories for Bayesian and variational inverse problems”.
Published on Friday 28 February 2020 at 12:00 UTC #group #job #dfg #map-estimators #ayanbayev