#ouq
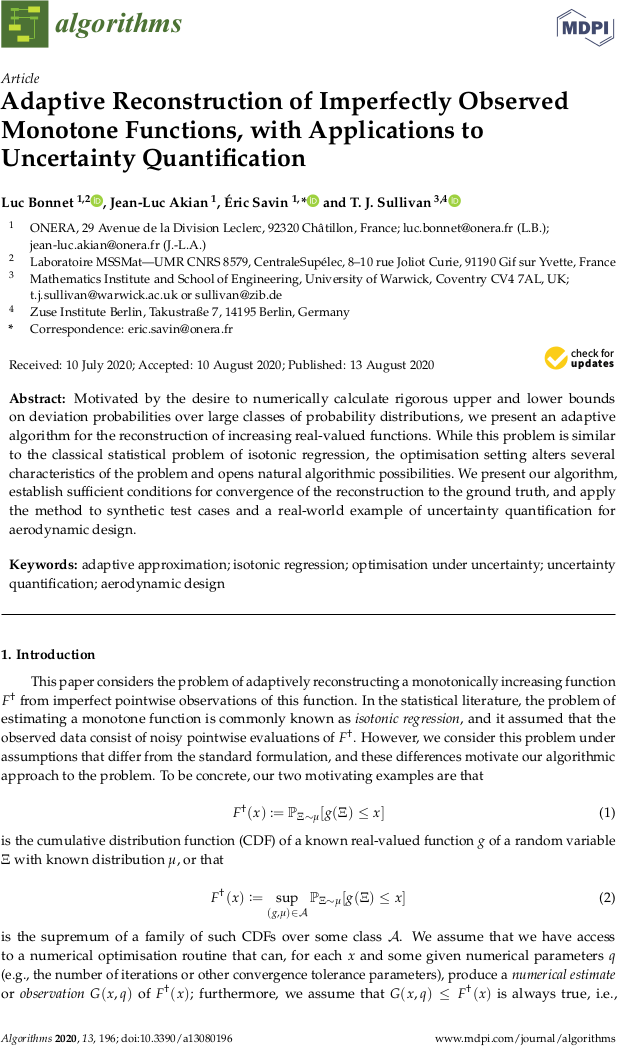
Adaptive reconstruction of monotone functions in special issue of Algorithms
The paper “Adaptive reconstruction of imperfectly-observed monotone functions, with applications to uncertainty quantification” by Luc Bonnet, Jean-Luc Akian, Éric Savin, and myself has just appeared in a special issue of the journal Algorithms devoted to Methods and Applications of Uncertainty Quantification in Engineering and Science. In this work, motivated by the computational needs of the optimal uncertainty quantification (OUQ) framework, we present and develop an algorithm for reconstructing a monotone function \(F\) given the ability to interrogate \(F\) pointwise but subject to partially controllable one-sided observational errors of the type that one would typically encounter if the observations would arise from a numerical optimisation routine.
L. Bonnet, J.-L. Akian, É. Savin, and T. J. Sullivan. “Adaptive reconstruction of imperfectly-observed monotone functions, with applications to uncertainty quantification.” Algorithms 13(8):no. 196, 21pp., 2020.
Abstract. Motivated by the desire to numerically calculate rigorous upper and lower bounds on deviation probabilities over large classes of probability distributions, we present an adaptive algorithm for the reconstruction of increasing real-valued functions. While this problem is similar to the classical statistical problem of isotonic regression, the optimisation setting alters several characteristics of the problem and opens natural algorithmic possibilities. We present our algorithm, establish sufficient conditions for convergence of the reconstruction to the ground truth, and apply the method to synthetic test cases and a real-world example of uncertainty quantification for aerodynamic design.
Published on Monday 17 August 2020 at 12:00 UTC #publication #algorithms #daad #ouq #isotonic #bonnet #akian #savin
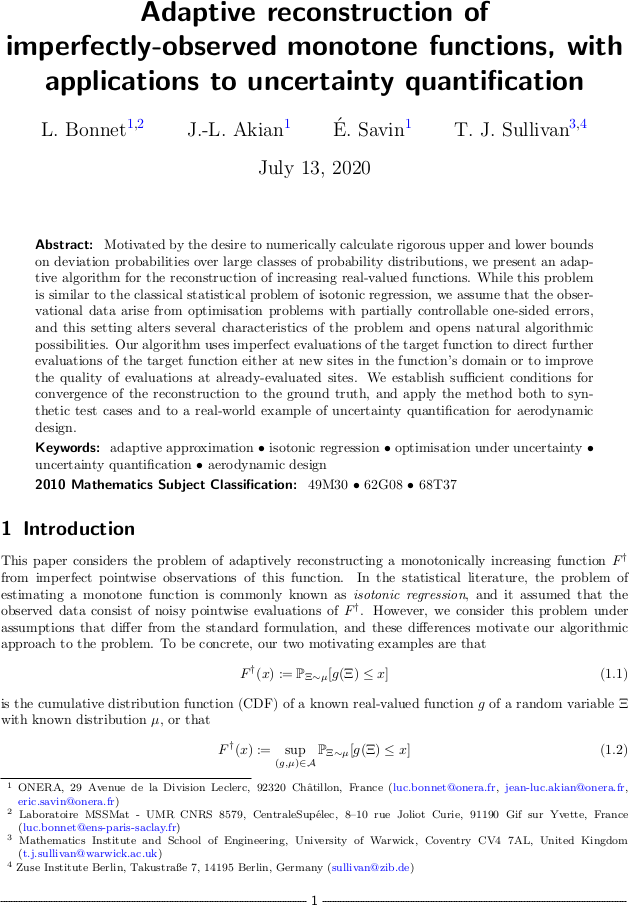
Adaptive reconstruction of monotone functions
Luc Bonnet, Jean-Luc Akian, Éric Savin, and I have just uploaded a preprint of our recent work “Adaptive reconstruction of imperfectly-observed monotone functions, with applications to uncertainty quantification” to the arXiv. In this work, motivated by the computational needs of the optimal uncertainty quantification (OUQ) framework, we present and develop an algorithm for reconstructing a monotone function \(F\) given the ability to interrogate \(F\) pointwise but subject to partially controllable one-sided observational errors of the type that one would typically encounter if the observations would arise from a numerical optimisation routine.
Abstract. Motivated by the desire to numerically calculate rigorous upper and lower bounds on deviation probabilities over large classes of probability distributions, we present an adaptive algorithm for the reconstruction of increasing real-valued functions. While this problem is similar to the classical statistical problem of isotonic regression, we assume that the observational data arise from optimisation problems with partially controllable one-sided errors, and this setting alters several characteristics of the problem and opens natural algorithmic possibilities. Our algorithm uses imperfect evaluations of the target function to direct further evaluations of the target function either at new sites in the function's domain or to improve the quality of evaluations at already-evaluated sites. We establish sufficient conditions for convergence of the reconstruction to the ground truth, and apply the method both to synthetic test cases and to a real-world example of uncertainty quantification for aerodynamic design.
Published on Monday 13 July 2020 at 10:00 UTC #preprint #daad #ouq #isotonic #bonnet #akian #savin
Luc Bonnet visits the UQ Group
It is a pleasure to announce that Luc Bonnet will be joining the UQ research group for a three-month visit funded by the German Academic Exchange Service (DAAD), with effect from 1 September 2019. He will be working on the application of optimal uncertainty quantification methodology to problems in aerodynamics and aircraft design.
Published on Sunday 1 September 2019 at 09:00 UTC #group #daad #ouq #bonnet
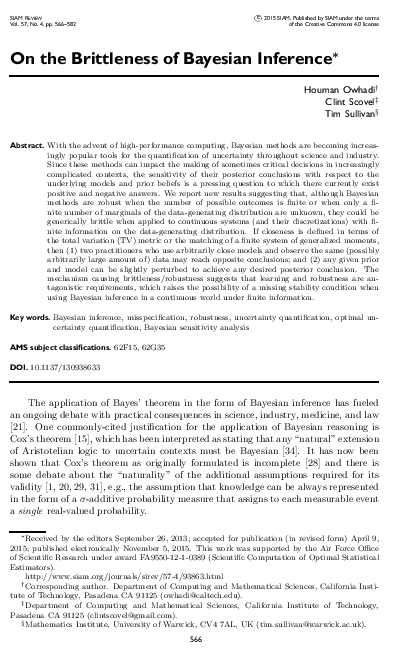
Bayesian Brittleness in SIAM Review
The 2015 Q4 issue of SIAM Review will carry an article by Houman Owhadi, Clint Scovel, and myself on the brittle dependency of Bayesian posteriors as a function of the prior. This is an abbreviated presentation of results given in full earlier this year in Elec. J. Stat. The PDF is available for free under the terms of the Creative Commons 4.0 licence.
H. Owhadi, C. Scovel, and T. J. Sullivan. “On the brittleness of Bayesian inference.” SIAM Review 57(4):566–582, 2015.
Abstract. With the advent of high-performance computing, Bayesian methods are becoming increasingly popular tools for the quantification of uncertainty throughout science and industry. Since these methods can impact the making of sometimes critical decisions in increasingly complicated contexts, the sensitivity of their posterior conclusions with respect to the underlying models and prior beliefs is a pressing question to which there currently exist positive and negative answers. We report new results suggesting that, although Bayesian methods are robust when the number of possible outcomes is finite or when only a finite number of marginals of the data-generating distribution are unknown, they could be generically brittle when applied to continuous systems (and their discretizations) with finite information on the data-generating distribution. If closeness is defined in terms of the total variation (TV) metric or the matching of a finite system of generalized moments, then (1) two practitioners who use arbitrarily close models and observe the same (possibly arbitrarily large amount of) data may reach opposite conclusions; and (2) any given prior and model can be slightly perturbed to achieve any desired posterior conclusion. The mechanism causing brittleness/robustness suggests that learning and robustness are antagonistic requirements, which raises the possibility of a missing stability condition when using Bayesian inference in a continuous world under finite information.
Published on Friday 6 November 2015 at 12:00 UTC #publication #siam-review #ouq #bayesian #owhadi #scovel
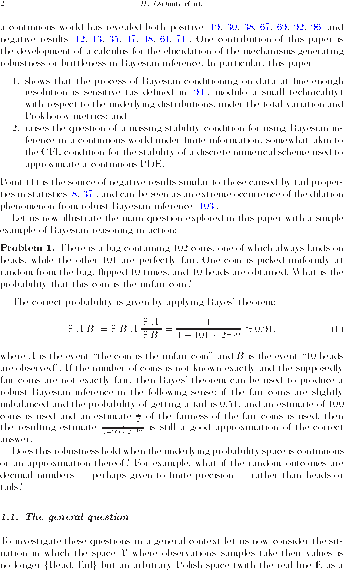
Bayesian Brittleness in Elec. J. Stat.
The Electronic Journal of Statistics has published an article by Houman Owhadi, Clint Scovel, and myself on the brittle dependency of Bayesian posteriors as a function of the prior.
H. Owhadi, C. Scovel, and T. J. Sullivan. “Brittleness of Bayesian inference under finite information in a continuous world.” Electronic Journal of Statistics 9(1):1–79, 2015.
Abstract. We derive, in the classical framework of Bayesian sensitivity analysis, optimal lower and upper bounds on posterior values obtained from Bayesian models that exactly capture an arbitrarily large number of finite-dimensional marginals of the data-generating distribution and/or that are as close as desired to the data-generating distribution in the Prokhorov or total variation metrics; these bounds show that such models may still make the largest possible prediction error after conditioning on an arbitrarily large number of sample data measured at finite precision. These results are obtained through the development of a reduction calculus for optimization problems over measures on spaces of measures. We use this calculus to investigate the mechanisms that generate brittleness/robustness and, in particular, we observe that learning and robustness are antagonistic properties. It is now well understood that the numerical resolution of PDEs requires the satisfaction of specific stability conditions. Is there a missing stability condition for using Bayesian inference in a continuous world under finite information?
Published on Tuesday 3 February 2015 at 10:00 UTC #publication #elec-j-stat #ouq #bayesian #owhadi #scovel