Welcome!
I am an Associate Professor in Predictive Modelling in the Mathematics Institute and School of Engineering at the University of Warwick. I have wide interests in uncertainty quantification in the broad sense, understood as the meeting point of numerical analysis, applied probability and statistics, and scientific computation. On this site you will find information about how to contact me, my research, publications, and teaching activities.
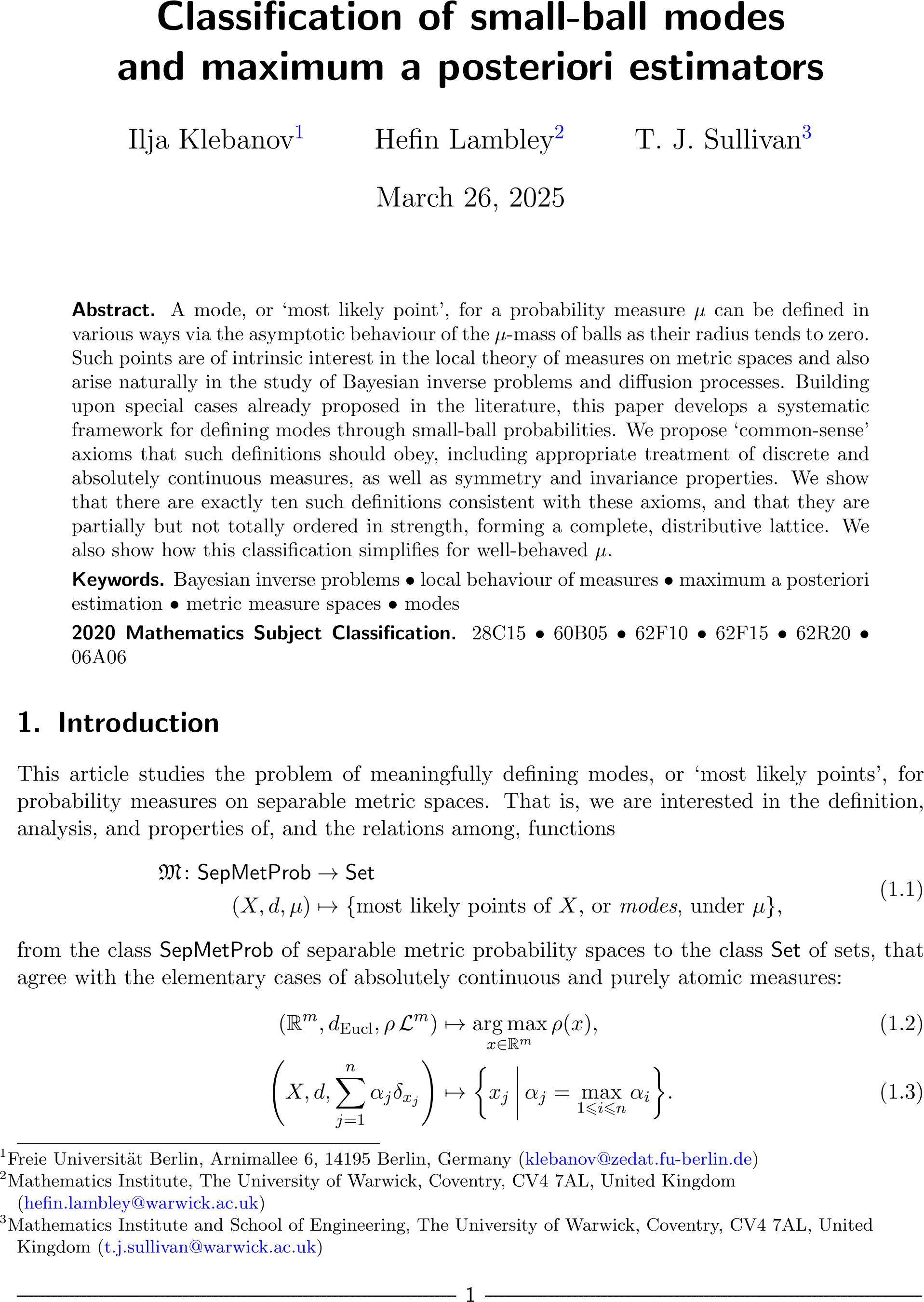
Classification of small-ball modes and maximum a posteriori estimators
Ilja Klebanov, Hefin Lambley, and I have just uploaded a preprint of our paper “Classification of small-ball modes and maximum a posteriori estimators” to the arXiv. This work thoroughly revises and extends our earlier preprint “A ‘periodic table’ of modes and maximum a posteriori estimators”.
Abstract. A mode, or “most likely point”, for a probability measure \(\mu\) can be defined in various ways via the asymptotic behaviour of the \(\mu\)-mass of balls as their radius tends to zero. Such points are of intrinsic interest in the local theory of measures on metric spaces and also arise naturally in the study of Bayesian inverse problems and diffusion processes. Building upon special cases already proposed in the literature, this paper develops a systematic framework for defining modes through small-ball probabilities. We propose “common-sense” axioms that such definitions should obey, including appropriate treatment of discrete and absolutely continuous measures, as well as symmetry and invariance properties. We show that there are exactly ten such definitions consistent with these axioms, and that they are partially but not totally ordered in strength, forming a complete, distributive lattice. We also show how this classification simplifies for well-behaved \(\mu\).
Published on Wednesday 26 March 2025 at 12:00 UTC #preprint #modes #map-estimators #klebanov #lambley
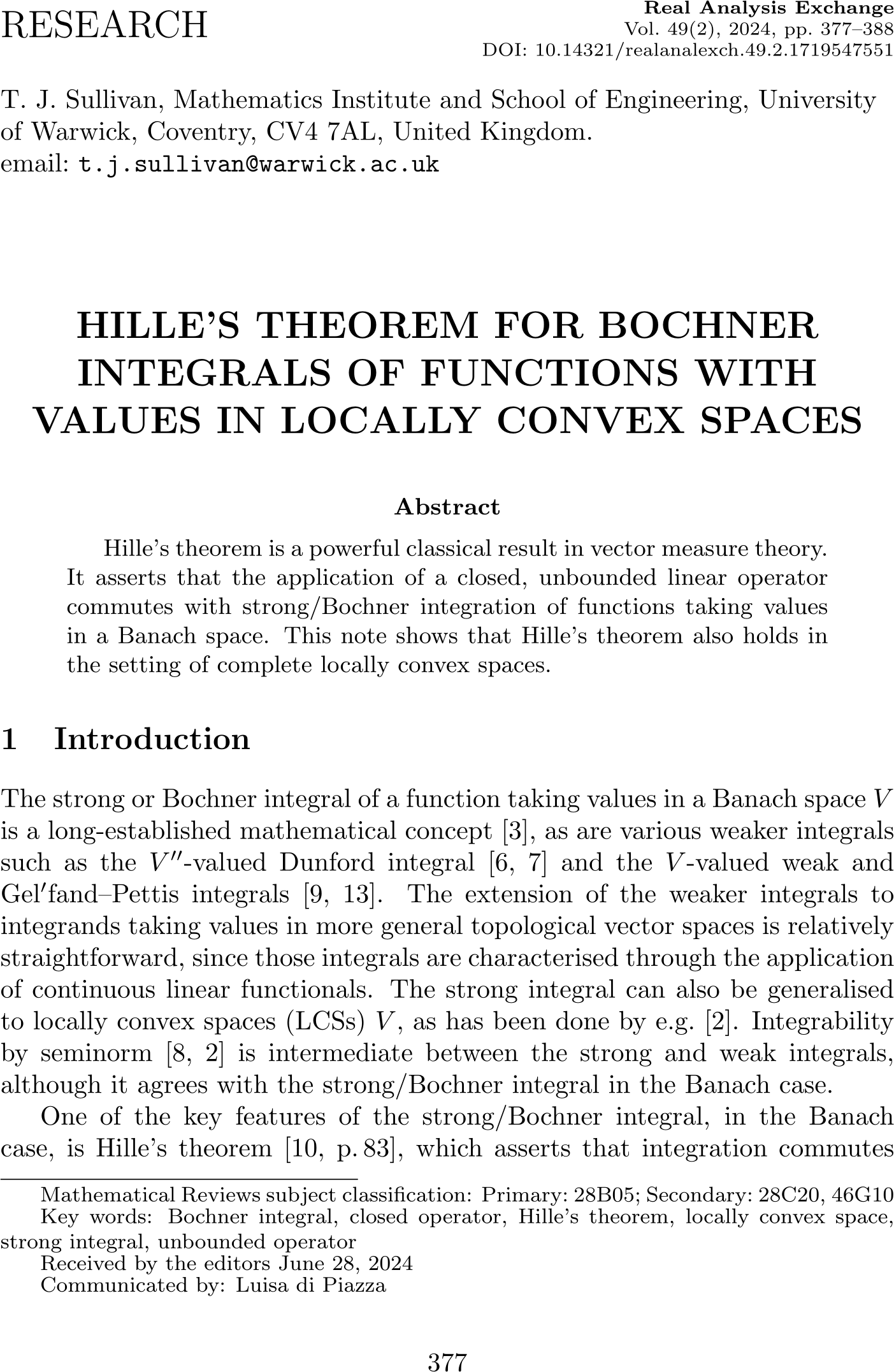
Hille's theorem for locally convex spaces in Real Analysis Exchange
The article “Hille's theorem for Bochner integrals of functions with values in locally convex spaces” has just appeared in its final form in Real Analysis Exchange.
T. J. Sullivan. “Hille's theorem for Bochner integrals of functions with values in locally convex spaces.” Real Analysis Exchange 49(2):377–388, 2024.
Abstract. Hille's theorem is a powerful classical result in vector measure theory. It asserts that the application of a closed, unbounded linear operator commutes with strong/Bochner integration of functions taking values in a Banach space. This note shows that Hille's theorem also holds in the setting of complete locally convex spaces.
Published on Tuesday 1 October 2024 at 13:00 UTC #publication #real-anal-exch #functional-analysis #hille-theorem
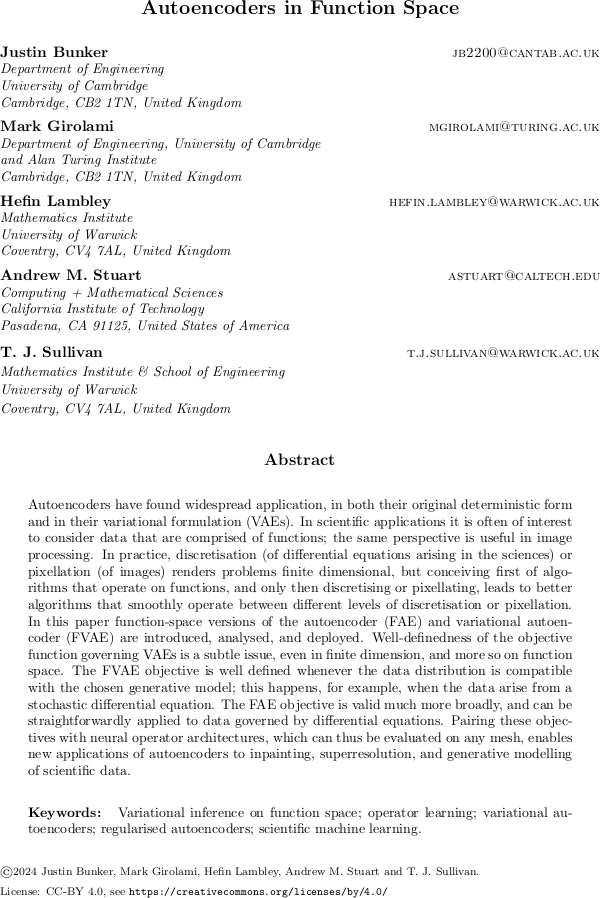
Autoencoders in function space
Justin Bunker, Mark Girolami, Hefin Lambley, Andrew Stuart and I have just uploaded a preprint of our paper “Autoencoders in function space” to the arXiv.
Abstract. Autoencoders have found widespread application, in both their original deterministic form and in their variational formulation (VAEs). In scientific applications it is often of interest to consider data that are comprised of functions; the same perspective is useful in image processing. In practice, discretisation (of differential equations arising in the sciences) or pixellation (of images) renders problems finite dimensional, but conceiving first of algorithms that operate on functions, and only then discretising or pixellating, leads to better algorithms that smoothly operate between different levels of discretisation or pixellation. In this paper function-space versions of the autoencoder (FAE) and variational autoencoder (FVAE) are introduced, analysed, and deployed. Well-definedness of the objective function governing VAEs is a subtle issue, even in finite dimension, and more so on function space. The FVAE objective is well defined whenever the data distribution is compatible with the chosen generative model; this happens, for example, when the data arise from a stochastic differential equation. The FAE objective is valid much more broadly, and can be straightforwardly applied to data governed by differential equations. Pairing these objectives with neural operator architectures, which can thus be evaluated on any mesh, enables new applications of autoencoders to inpainting, superresolution, and generative modelling of scientific data.
Published on Monday 5 August 2024 at 12:00 UTC #preprint #bunker #girolami #lambley #stuart #autoencoders
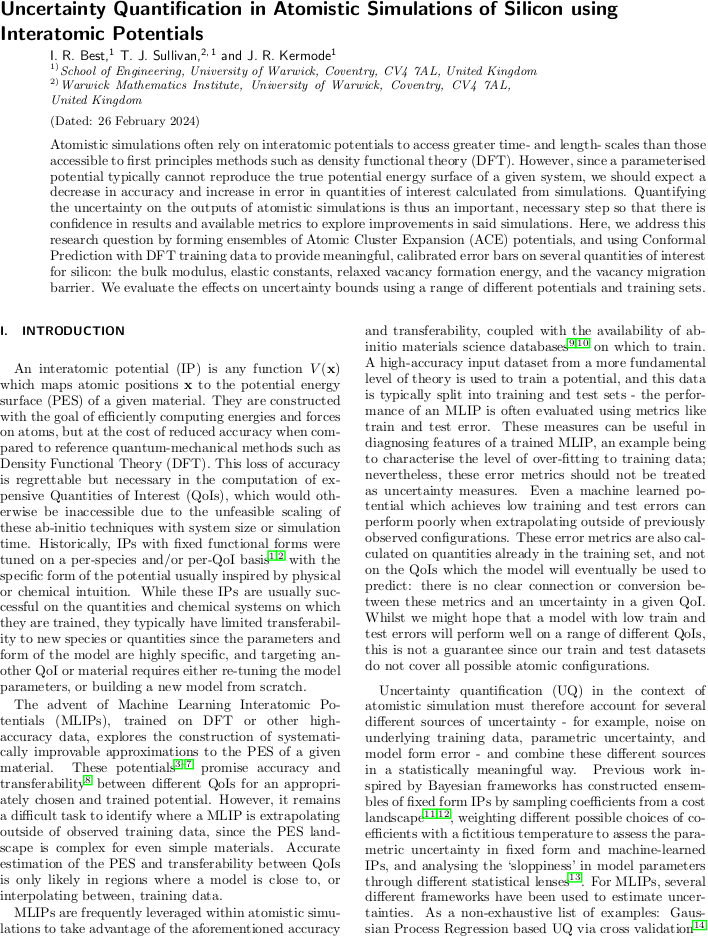
UQ for Si using interatomic potentials
Iain Best, James Kermode, and I have just uploaded a preprint of our paper “Uncertainty quantification in atomistic simulations of silicon using interatomic potentials” to the arXiv.
Abstract. Atomistic simulations often rely on interatomic potentials to access greater time- and length- scales than those accessible to first principles methods such as density functional theory (DFT). However, since a parameterised potential typically cannot reproduce the true potential energy surface of a given system, we should expect a decrease in accuracy and increase in error in quantities of interest calculated from simulations. Quantifying the uncertainty on the outputs of atomistic simulations is thus an important, necessary step so that there is confidence in results and available metrics to explore improvements in said simulations. Here, we address this research question by forming ensembles of Atomic Cluster Expansion (ACE) potentials, and using Conformal Prediction with DFT training data to provide meaningful, calibrated error bars on several quantities of interest for silicon: the bulk modulus, elastic constants, relaxed vacancy formation energy, and the vacancy migration barrier. We evaluate the effects on uncertainty bounds using a range of different potentials and training sets.
Published on Saturday 24 February 2024 at 12:00 UTC #preprint #kermode #best #uq #interatomc-potentials
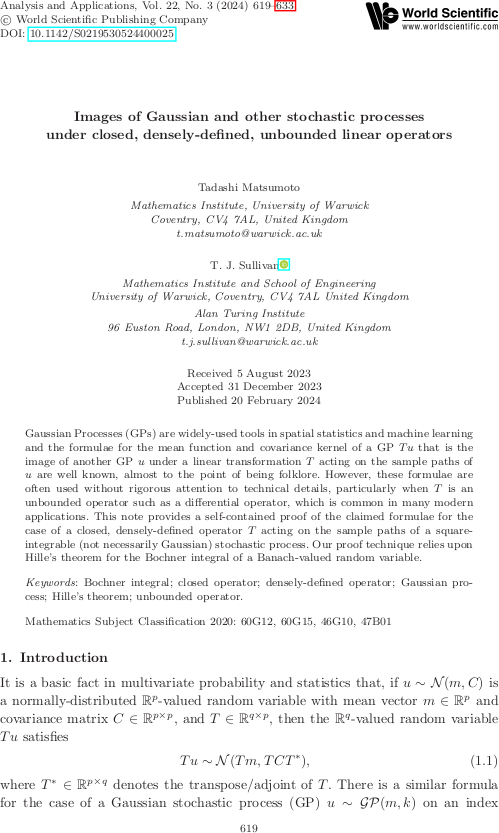
Unbounded images of Gaussian and other stochastic processes in Analysis and Applications
The final version of “Images of Gaussian and other stochastic processes under closed, densely-defined, unbounded linear operators” by Tadashi Matsumoto and myself has just appeared in Analysis and Applications.
The purpose of this article is to provide a self-contained rigorous proof of the well-known formula for the mean and covariance function of a stochastic process — in particular, a Gaussian process — when it is acted upon by an unbounded linear operator such as an ordinary or partial differential operator, as used in probabilistic approaches to the solution of ODEs and PDEs. This result is easy to establish in the case of a bounded operator, but the unbounded case requires a careful application of Hille's theorem for the Bochner integral of a Banach-valued random variable.
T. Matsumoto and T. J. Sullivan. “Images of Gaussian and other stochastic processes under closed, densely-defined, unbounded linear operators.” Analysis and Applications 22(3):619–633, 2024.
Abstract. Gaussian processes (GPs) are widely-used tools in spatial statistics and machine learning and the formulae for the mean function and covariance kernel of a GP \(v\) that is the image of another GP \(u\) under a linear transformation \(T\) acting on the sample paths of \(u\) are well known, almost to the point of being folklore. However, these formulae are often used without rigorous attention to technical details, particularly when \(T\) is an unbounded operator such as a differential operator, which is common in several modern applications. This note provides a self-contained proof of the claimed formulae for the case of a closed, densely-defined operator \(T\) acting on the sample paths of a square-integrable stochastic process. Our proof technique relies upon Hille's theorem for the Bochner integral of a Banach-valued random variable.
Published on Wednesday 21 February 2024 at 10:00 UTC #publication #anal-appl #prob-num #gp #matsumoto