#kermode
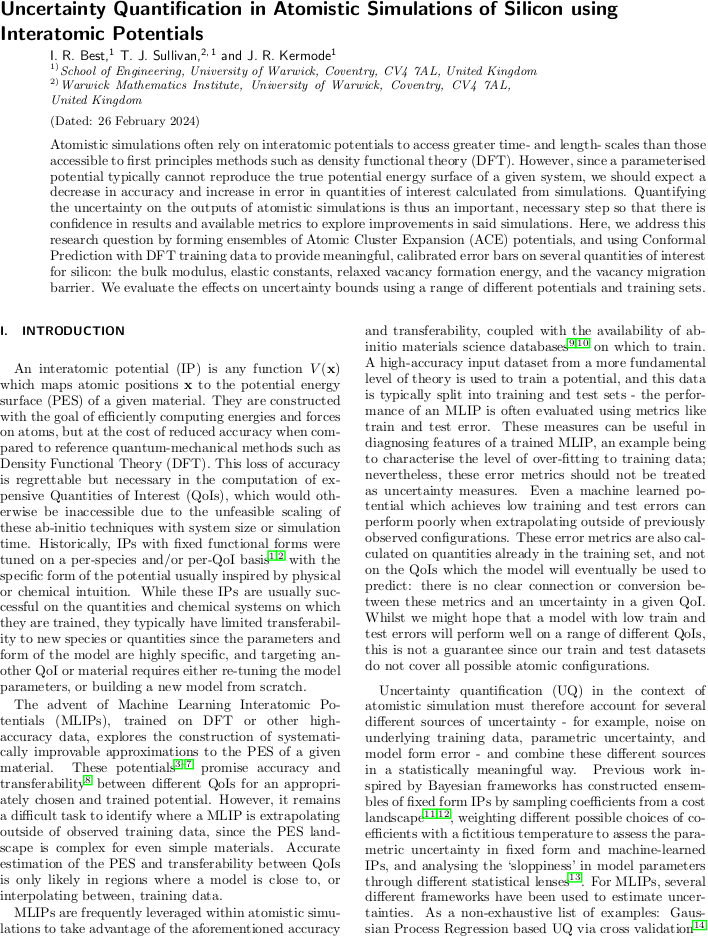
UQ for Si using interatomic potentials
Iain Best, James Kermode, and I have just uploaded a preprint of our paper “Uncertainty quantification in atomistic simulations of silicon using interatomic potentials” to the arXiv.
Abstract. Atomistic simulations often rely on interatomic potentials to access greater time- and length- scales than those accessible to first principles methods such as density functional theory (DFT). However, since a parameterised potential typically cannot reproduce the true potential energy surface of a given system, we should expect a decrease in accuracy and increase in error in quantities of interest calculated from simulations. Quantifying the uncertainty on the outputs of atomistic simulations is thus an important, necessary step so that there is confidence in results and available metrics to explore improvements in said simulations. Here, we address this research question by forming ensembles of Atomic Cluster Expansion (ACE) potentials, and using Conformal Prediction with DFT training data to provide meaningful, calibrated error bars on several quantities of interest for silicon: the bulk modulus, elastic constants, relaxed vacancy formation energy, and the vacancy migration barrier. We evaluate the effects on uncertainty bounds using a range of different potentials and training sets.
Published on Saturday 24 February 2024 at 12:00 UTC #preprint #kermode #best #uq #interatomc-potentials
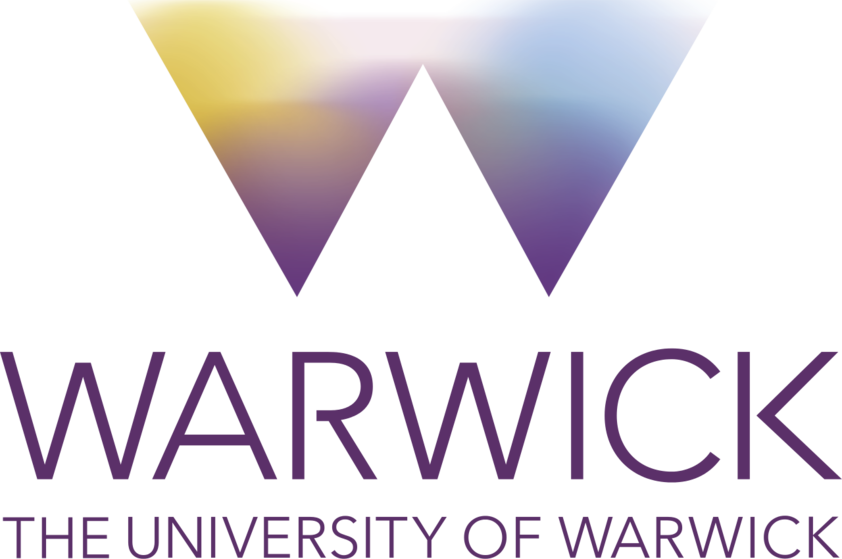
PhD Project on Adaptive Probabilistic Meshless Methods
There is an opening for a PhD student to work with me and co-PIs Jon Cockayne and James Kermode on the project “Adaptive probabilistic meshless methods for evolutionary systems” as part of the EPSRC Centre for Doctoral Training in Modelling of Heterogeneous Systems at the University of Warwick.
This project will develop and implement a new class of numerical solvers for evolving systems such as interacting fluid-structure flows. To cope with extreme strain rates and large deformations these new solvers will be adaptive and meshless, and they will also implicitly represent their own solution uncertainty, thus enabling optimal design and uncertainty quantification. This exciting project brings together aspects of continuum mechanics, numerical methods for partial differential equations, and statistical machine learning.
Interested students should contact me and the other PIs with informal queries. Formal applications should use the HetSys application page.
Published on Monday 20 April 2020 at 08:00 UTC #group #job #phd #warwick #hetsys #kermode #cockayne