#girolami
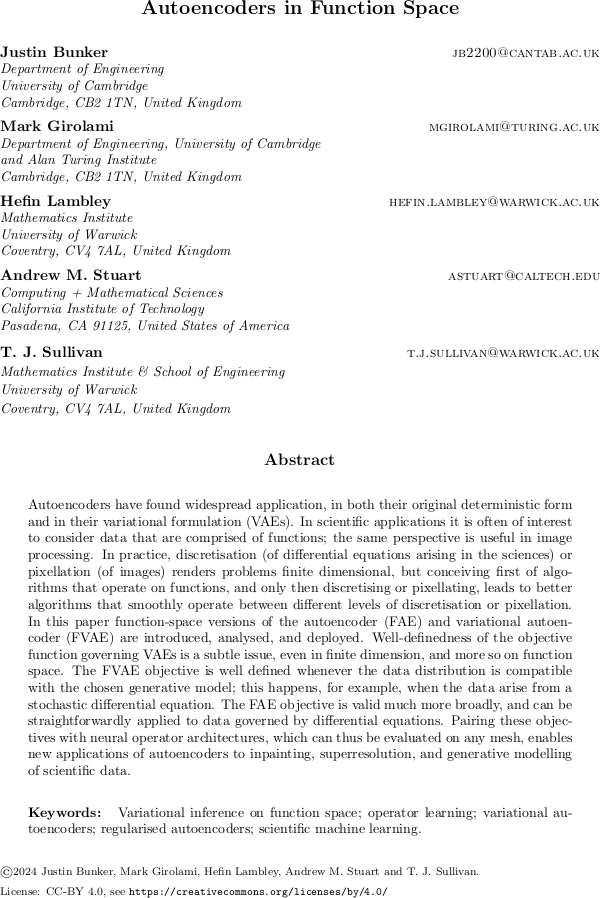
Autoencoders in function space
Justin Bunker, Mark Girolami, Hefin Lambley, Andrew Stuart and I have just uploaded a preprint of our paper “Autoencoders in function space” to the arXiv.
Abstract. Autoencoders have found widespread application, in both their original deterministic form and in their variational formulation (VAEs). In scientific applications it is often of interest to consider data that are comprised of functions; the same perspective is useful in image processing. In practice, discretisation (of differential equations arising in the sciences) or pixellation (of images) renders problems finite dimensional, but conceiving first of algorithms that operate on functions, and only then discretising or pixellating, leads to better algorithms that smoothly operate between different levels of discretisation or pixellation. In this paper function-space versions of the autoencoder (FAE) and variational autoencoder (FVAE) are introduced, analysed, and deployed. Well-definedness of the objective function governing VAEs is a subtle issue, even in finite dimension, and more so on function space. The FVAE objective is well defined whenever the data distribution is compatible with the chosen generative model; this happens, for example, when the data arise from a stochastic differential equation. The FAE objective is valid much more broadly, and can be straightforwardly applied to data governed by differential equations. Pairing these objectives with neural operator architectures, which can thus be evaluated on any mesh, enables new applications of autoencoders to inpainting, superresolution, and generative modelling of scientific data.
Published on Monday 5 August 2024 at 12:00 UTC #preprint #bunker #girolami #lambley #stuart #autoencoders
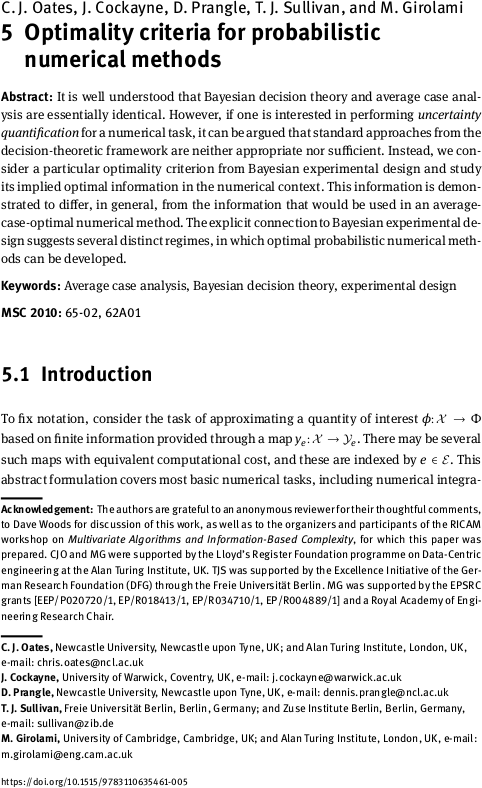
Optimality of probabilistic numerical methods
The paper “Optimality criteria for probabilistic numerical methods” by Chris Oates, Jon Cockayne, Dennis Prangle, Mark Girolami, and myself has just appeared in print:
C. J. Oates, J. Cockayne, D. Prangle, T. J. Sullivan, and M. Girolami. “Optimality criteria for probabilistic numerical methods” in Multivariate Algorithms and Information-Based Complexity, ed. F. J. Hickernell and P. Kritzer. Radon Series on Computational and Applied Mathematics 27:65–88, 2020.
Abstract. It is well understood that Bayesian decision theory and average case analysis are essentially identical. However, if one is interested in performing uncertainty quantification for a numerical task, it can be argued that standard approaches from the decision-theoretic framework are neither appropriate nor sufficient. Instead, we consider a particular optimality criterion from Bayesian experimental design and study its implied optimal information in the numerical context. This information is demonstrated to differ, in general, from the information that would be used in an average-case-optimal numerical method. The explicit connection to Bayesian experimental design suggests several distinct regimes, in which optimal probabilistic numerical methods can be developed.
Published on Sunday 31 May 2020 at 08:00 UTC #publication #prob-num #oates #cockayne #prangle #girolami
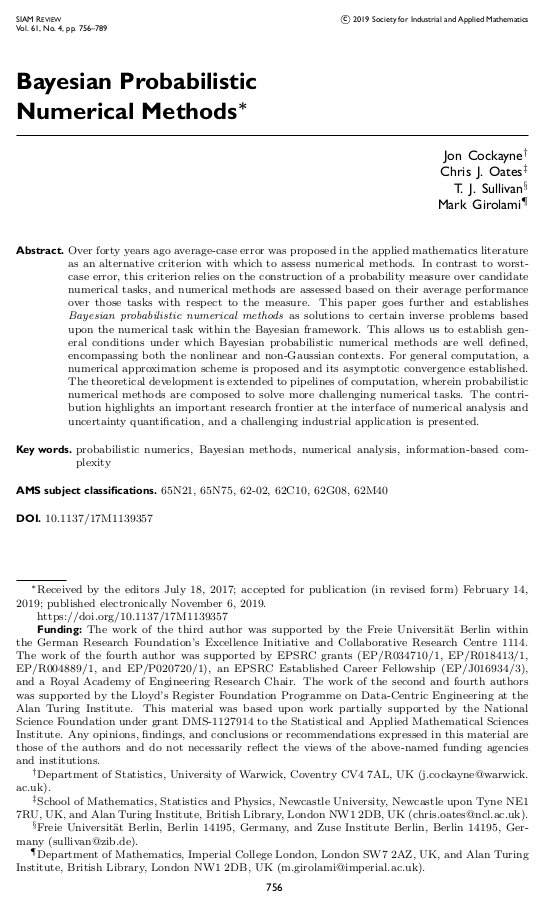
Bayesian probabilistic numerical methods in SIAM Review
The 2019 Q4 issue of SIAM Review will carry an article by Jon Cockayne, Chris Oates, Mark Girolami, and myself on the Bayesian formulation of probabilistic numerical methods, i.e. the interpretation of deterministic numerical tasks such as quadrature and the solution of ordinary and partial differential equations as (Bayesian) statistical inference tasks.
J. Cockayne, C. J. Oates, T. J. Sullivan, and M. Girolami. “Bayesian probabilistic numerical methods.” SIAM Review 61(4):756–789, 2019.
Abstract. Over forty years ago average-case error was proposed in the applied mathematics literature as an alternative criterion with which to assess numerical methods. In contrast to worst-case error, this criterion relies on the construction of a probability measure over candidate numerical tasks, and numerical methods are assessed based on their average performance over those tasks with respect to the measure. This paper goes further and establishes Bayesian probabilistic numerical methods as solutions to certain inverse problems based upon the numerical task within the Bayesian framework. This allows us to establish general conditions under which Bayesian probabilistic numerical methods are well defined, encompassing both the nonlinear and non-Gaussian contexts. For general computation, a numerical approximation scheme is proposed and its asymptotic convergence established. The theoretical development is extended to pipelines of computation, wherein probabilistic numerical methods are composed to solve more challenging numerical tasks. The contribution highlights an important research frontier at the interface of numerical analysis and uncertainty quantification, and a challenging industrial application is presented.
Published on Thursday 7 November 2019 at 07:00 UTC #publication #bayesian #siam-review #prob-num #cockayne #girolami #oates
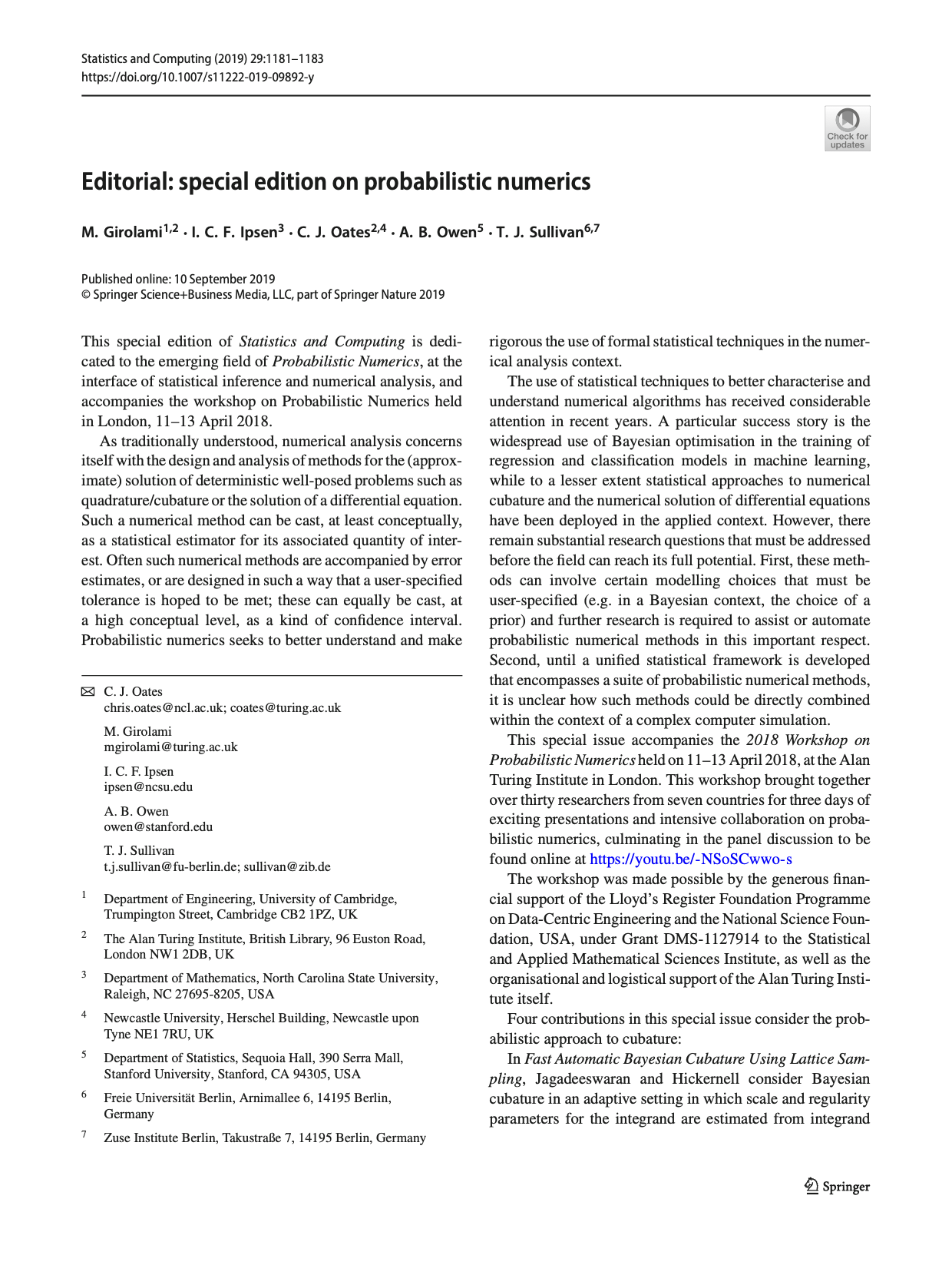
Statistics and Computing special issue on Probabilistic Numerics
It is a great pleasure to announce that a special issue of Statistics and Computing (vol. 29, no. 6) dedicated to the theme of probabilistic numerics is now fully available online in print. This special issue, edited by Mark Girolami, Ilse Ipsen, Chris Oates, Art Owen, and myself, accompanies the 2018 Workshop on Probabilistic Numerics held at the Alan Turing Institute in London.
The special issue consists of a short editorial and ten full-length peer-reviewed research articles:
- “De-noising by thresholding operator adapted wavelets” by G. R. Yoo and H. Owhadi
- “Optimal Monte Carlo integration on closed manifolds” by M. Ehler, M. Gräf, and C. J. Oates
- “Fast automatic Bayesian cubature using lattice sampling” by R. Jagadeeswaran and F. J. Hickernell
- “Symmetry exploits for Bayesian cubature methods” by T. Karvonen, S. Särkkä, and C. J. Oates
- “Probabilistic linear solvers: a unifying view” by S. Bartels, J. Cockayne, I. C. F. Ipsen, and P. Hennig
- “Strong convergence rates of probabilistic integrators for ordinary differential equations” by H. C. Lie, A. M. Stuart, and T. J. Sullivan
- “Adaptive step-size selection for state-space based probabilistic differential equation solvers” by O. A. Chkrebtii and D. A. Campbell
- “Probabilistic solutions to ordinary differential equations as non-linear Bayesian filtering: A new perspective” by F. Tronarp, H. Kersting, S. Särkkä, and P. Hennig
- “On the positivity and magnitudes of Bayesian quadrature weights” by T. Karvonen, M. Kanagawa, and S. Särkkä
- “A modern retrospective on probabilistic numerics” by C. J. Oates and T. J. Sullivan
Published on Wednesday 30 October 2019 at 12:00 UTC #stco #prob-num #girolami #ipsen #oates #owen
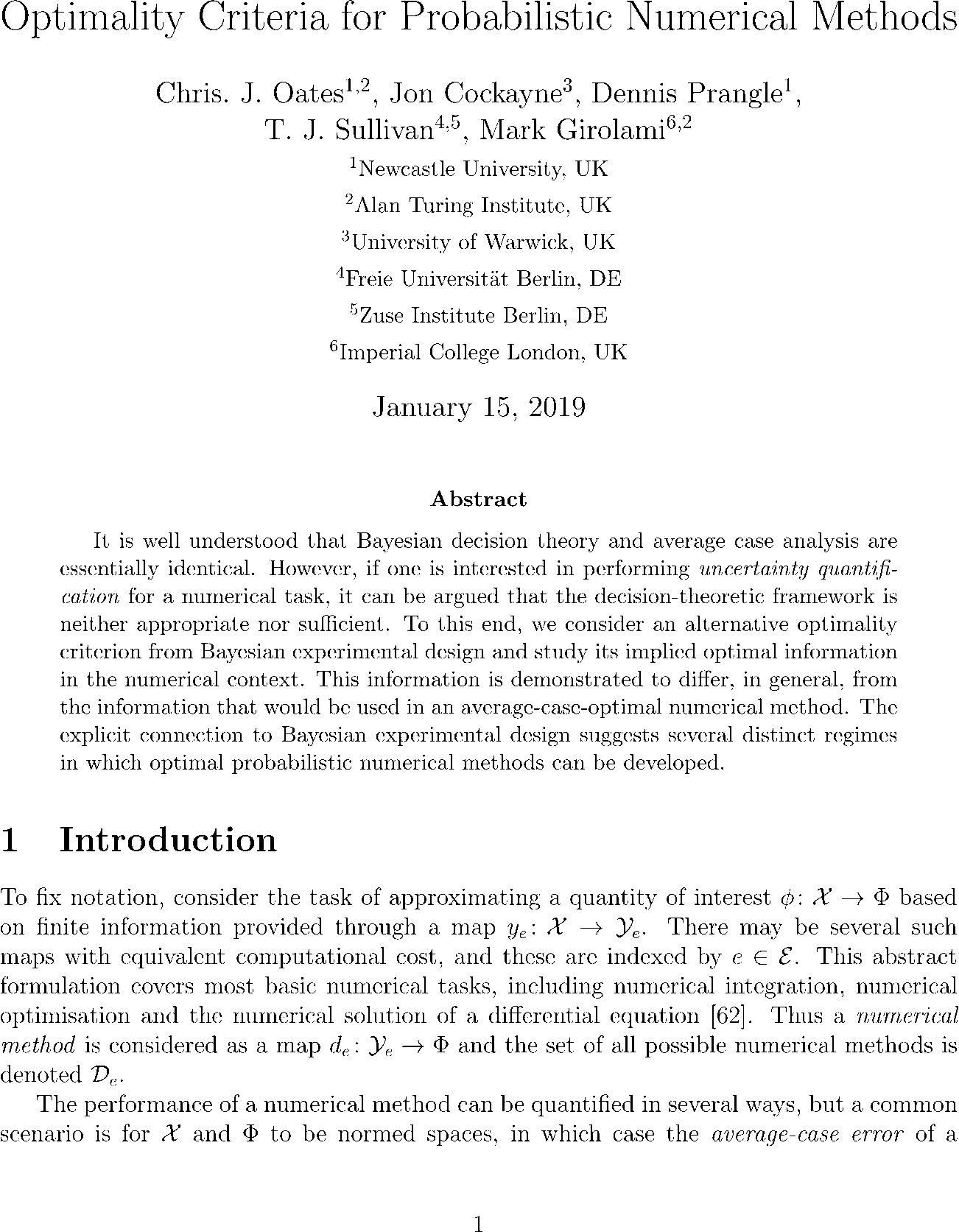
Optimality of probabilistic numerical methods
Chris Oates, Jon Cockayne, Dennis Prangle, Mark Girolami, and I have just uploaded a preprint of our paper “Optimality criteria for probabilistic numerical methods” to the arXiv.
Abstract. It is well understood that Bayesian decision theory and average case analysis are essentially identical. However, if one is interested in performing uncertainty quantification for a numerical task, it can be argued that the decision-theoretic framework is neither appropriate nor sufficient. To this end, we consider an alternative optimality criterion from Bayesian experimental design and study its implied optimal information in the numerical context. This information is demonstrated to differ, in general, from the information that would be used in an average-case-optimal numerical method. The explicit connection to Bayesian experimental design suggests several distinct regimes in which optimal probabilistic numerical methods can be developed.
Published on Tuesday 15 January 2019 at 11:00 UTC #preprint #prob-num #oates #cockayne #prangle #girolami